The Vital Role of Medical Datasets for Machine Learning in Modern Business
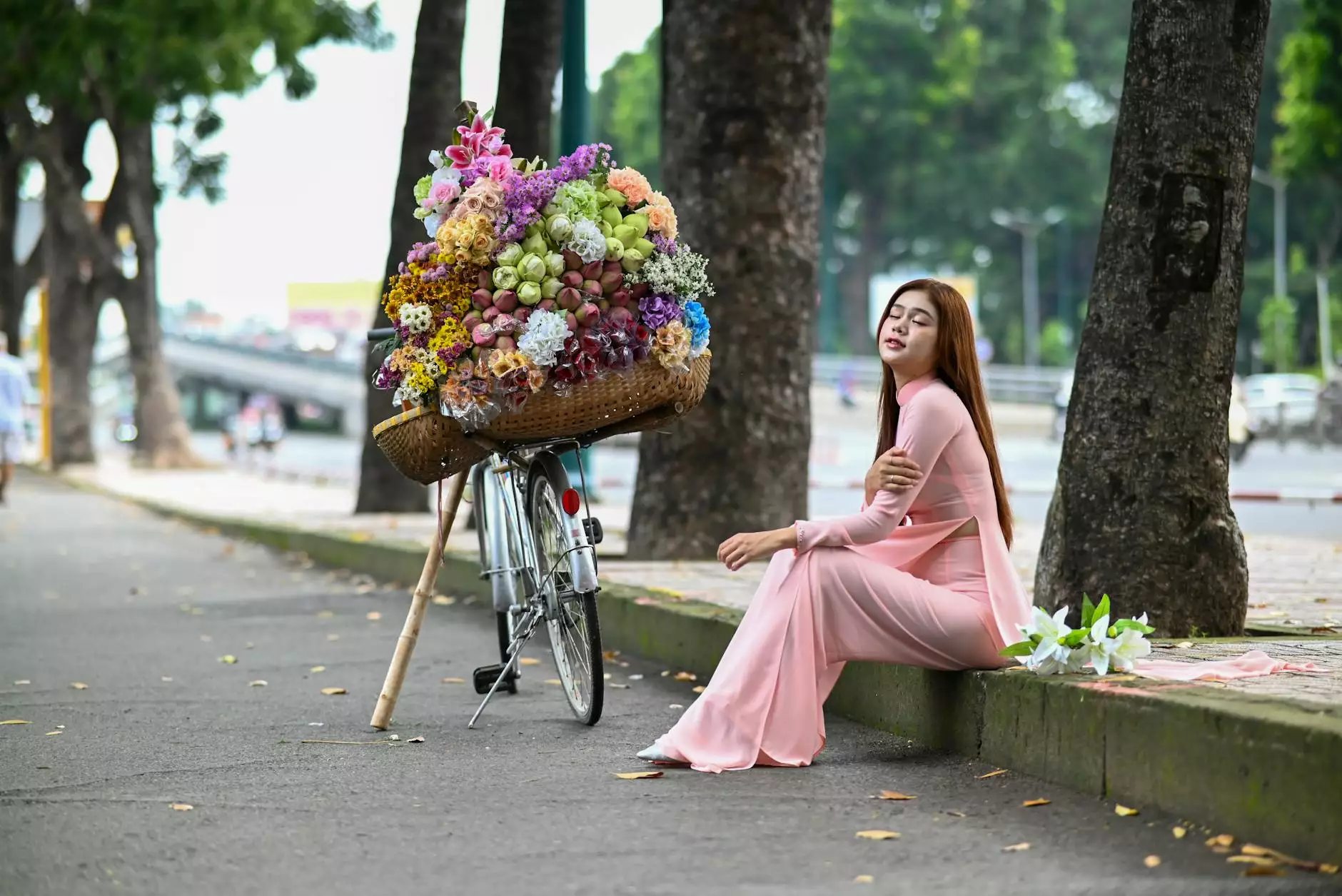
In today's fast-paced digital world, leveraging data is essential for the success of any business, especially in the health sector. The advent of machine learning (ML) has revolutionized how organizations operate, offering unprecedented opportunities for improving services, efficiency, and outcomes. Among the various data types that have emerged, the medical dataset for machine learning stands out as a cornerstone of innovation and advancement in healthcare.
Understanding Medical Datasets
A medical dataset comprises an organized collection of health-related data, which may include patient demographics, medical histories, treatments, outcomes, and various other clinical records. These datasets are critical in training machine learning models to achieve accuracy in predictions and decisions.
Types of Medical Datasets
- Clinical Trials Data: Data collected during clinical research, crucial for developing new treatments.
- Patient Records: Comprehensive information on individual patient histories and treatments.
- Genomic Data: Genetic information used for personalized medicine.
- Epidemiological Studies: Datasets that analyze health-related events across populations.
- Health Surveys: Data collected through questionnaires regarding health status and behaviors.
Each type of dataset serves unique purposes and offers different insights, contributing to the overall excellence in healthcare and associated businesses.
The Impact of Machine Learning on Healthcare
Machine learning, when combined with extensive medical datasets for machine learning, revolutionizes healthcare services. These technologies enable medical professionals and organizations to infer patterns, predict outcomes, and tailor treatments with remarkable accuracy. Some notable impacts include:
1. Improved Patient Outcomes
Applying machine learning algorithms to medical datasets enhances diagnostic accuracy and treatment efficacy. By analyzing historical data, ML models can identify potential health risks and recommend preventative measures, ultimately contributing to improved patient outcomes.
2. Predictive Analytics
Predictive analytics powered by machine learning helps healthcare providers forecast patient trends, such as potential health crises or the likelihood of Readmission. By understanding these patterns, businesses can effectively allocate resources and develop strategic interventions to mitigate risks.
3. Streamlined Operations
Organizations can automate various operational aspects with the insights gained from medical datasets. For example, advanced algorithms can optimize scheduling, manage inventories, and even predict staffing needs, allowing businesses to operate more efficiently.
4. Personalized Medicine
Personalized medicine is one of the most exciting developments resulting from machine learning applications in healthcare. By analyzing datasets pertaining to individual patient genetics, lifestyle, and environment, healthcare providers can tailor treatments to meet the specific needs of patients.
5. Cost Reduction
By utilizing machine learning and medical datasets, businesses can identify areas where costs can be reduced without sacrificing quality. Predictive maintenance of equipment and optimizing treatment plans lead to significant savings in both time and financial resources.
Harnessing the Power of Medical Datasets for Business Growth
For businesses operating in the healthcare sector, understanding and integrating medical datasets for machine learning into their operational strategies can unlock immense potentials. Here are essential strategies to leverage these datasets:
1. Building Comprehensive Data Infrastructure
A robust data infrastructure forms the backbone of any data-driven strategy. Organizations must invest in developing a comprehensive data management system to ensure that data is collected, stored, and analyzed efficiently.
2. Collaborating with Data Experts
Developing a strong partnership with data scientists and machine learning experts is crucial. These professionals have the skills to extract meaningful insights from medical datasets, turning raw data into strategic opportunities for growth.
3. Continuous Learning and Adaptation
Machine learning is an ever-evolving field. Organizations must continuously learn about the latest advancements and adapt their strategies accordingly. Regular training and updates will help ensure that businesses stay competitive.
4. Fostering a Culture of Data-Driven Decision-Making
Encouraging a culture that values data-driven decision-making is vital. Employees at all levels should understand the importance of data and be empowered to leverage it in their workflows.
Case Studies: Success Stories in Leveraging Medical Datasets
Numerous organizations have successfully harnessed medical datasets for machine learning. Here are a few noteworthy examples:
1. IBM Watson Health
IBM Watson Health utilizes machine learning on vast medical datasets to assist healthcare providers in cancer treatment. The platform analyzes patient data alongside medical literature to recommend personalized treatment options, significantly improving patient care.
2. Tempus
Tempus is a technology company that uses machine learning and data analytics to advance precision medicine. By analyzing clinical and molecular data, Tempus helps oncologists make data-driven decisions, enhancing treatment plans tailored for individual patients.
3. Google Health
Google Health is at the forefront of using machine learning and image recognition technologies to analyze medical images and detect conditions like diabetic retinopathy and breast cancer, showcasing the transformative power of medical datasets.
Challenges in Using Medical Datasets
Despite the significant advantages, several challenges are associated with utilizing medical datasets for machine learning. These include:
1. Data Privacy Concerns
Working with sensitive patient data raises privacy concerns. Organizations must comply with regulations such as HIPAA and ensure that robust data protection measures are in place.
2. Data Quality and Standardization
Poor-quality data can lead to misleading results. Ensuring that data is accurate, complete, and standardized is crucial for the success of machine learning projects.
3. Integration of Diverse Data Sources
Datasets often come from different sources and in various formats. Integrating these diverse data sources while maintaining data integrity can be challenging.
4. Skill Gaps in the Workforce
There is a growing demand for skilled professionals who can navigate the complex landscape of machine learning and healthcare analytics. Attracting and retaining talent can be a challenge for businesses.
Future Trends in Medical Datasets and Machine Learning
The intersection of medical datasets and machine learning continues to evolve, promising exciting developments in the healthcare sector. Some anticipated future trends include:
1. Enhanced Data Interoperability
Efforts to standardize medical data formats will facilitate smoother data sharing across different healthcare systems, ultimately enhancing the scope for machine learning applications.
2. AI-Driven Diagnostics
As machine learning models become more sophisticated, AI-driven diagnostic tools will likely become commonplace, leading to faster and more accurate diagnosis, improving overall healthcare quality.
3. Greater Focus on Social Determinants of Health
Integrating social determinants of health into datasets will enable more comprehensive analyses, offering insights into how various factors affect health outcomes and allowing for more effective interventions.
4. Real-World Evidence Generation
Machine learning will play a significant role in generating real-world evidence to inform regulatory decisions, treatment guidelines, and insurance reimbursement processes.
Conclusion
In conclusion, the utilization of medical datasets for machine learning is reshaping healthcare and associated businesses' operational landscape. Organizations that embrace this technological advancement stand to gain significantly in terms of improved patient care, operational efficiency, and long-term growth. By investing in comprehensive data strategies, fostering a culture of data-driven decision-making, and overcoming the challenges associated with data use, businesses can position themselves at the forefront of innovation in healthcare.
Keymakr.com is dedicated to utilizing these insights for its home services related to keys and locksmiths, demonstrating how the principles of machine learning can lead to superior customer service and operational excellence.