The Importance of Bounding Boxes in Data Annotation
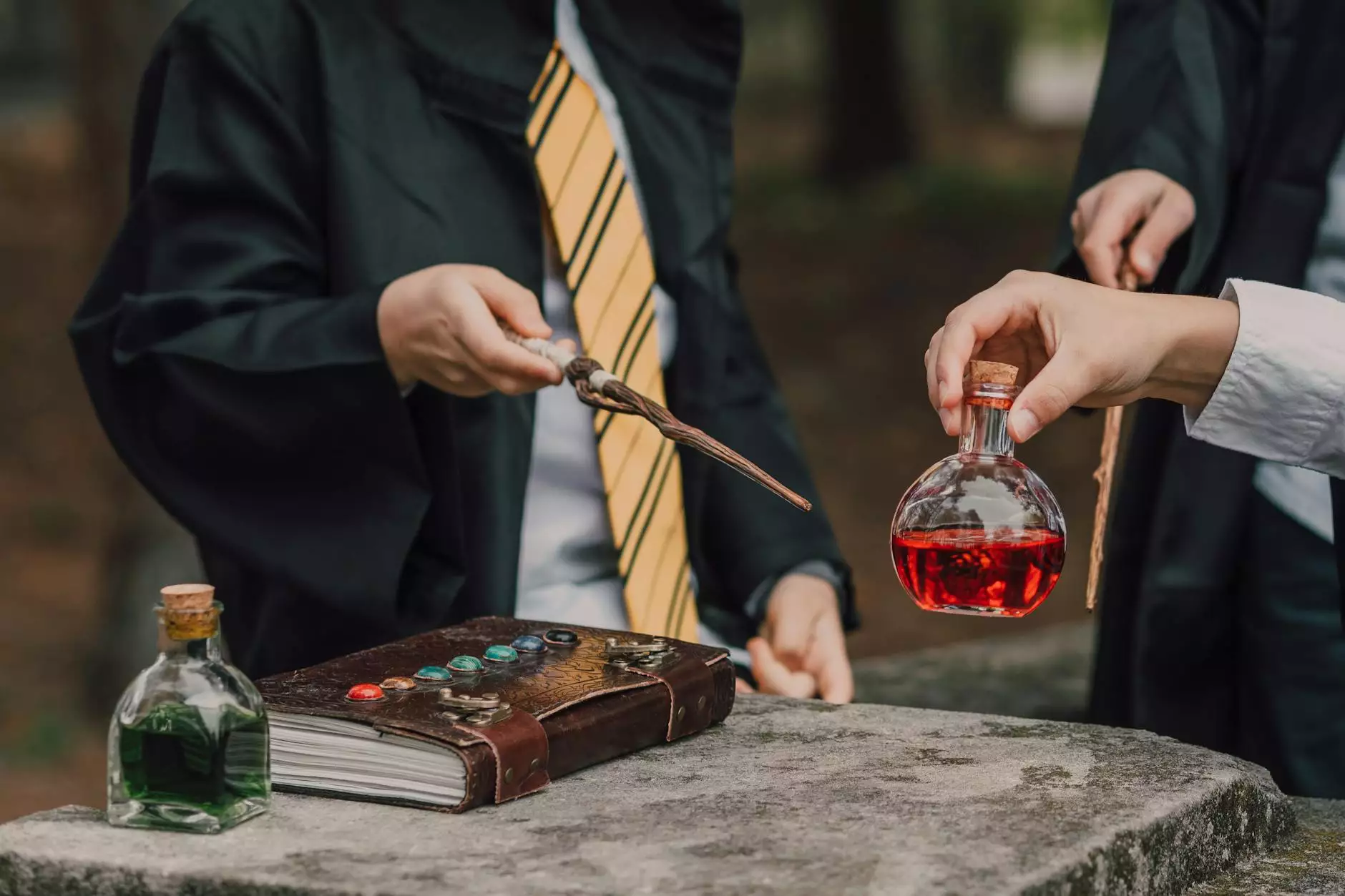
In the evolving landscape of technology, bounding boxes have emerged as a foundational element across numerous fields. Primarily utilized in computer vision, image processing, and machine learning, bounding boxes serve as rectangular indicators around objects within images, defining their position and extent. This article delves into the vital role of bounding boxes in data annotation, their applications in AI and machine learning, and how businesses like Keylabs.ai are utilizing these tools to enhance efficiency and accuracy.
Understanding Bounding Boxes
At its core, a bounding box is defined by two coordinates that outline the corners of the rectangle surrounding a target object. These coordinates provide essential data on the object's location within a larger frame. The importance of bounding boxes cannot be overstated; they enable algorithms to process visual data effectively, facilitating a wide range of applications that transform how machines interpret images.
Types of Bounding Boxes
Bounding boxes can be classified into three main types:
- 2D Bounding Boxes: The most common type, used in image processing applications to highlight objects in traditional two-dimensional images.
- 3D Bounding Boxes: Employed in applications requiring depth perception, such as in video games, simulations, and robotics. These boxes provide a volumetric representation of an object's boundaries.
- Rotated Bounding Boxes: Some objects are not perfectly rectangular, and rotated bounding boxes provide a more accurate representation by allowing for angular rotations.
Applications of Bounding Boxes
Bounding boxes find extensive applications in numerous domains:
1. Image Recognition
In image recognition tasks, bounding boxes assist in identifying and classifying objects within photos. They allow algorithms to concentrate on specific regions of an image rather than analyzing the entire picture, enhancing processing speed and accuracy.
2. Autonomous Vehicles
Autonomous driving systems heavily rely on bounding boxes for detecting pedestrians, traffic signs, vehicles, and obstacles. By providing real-time location data, these systems can make informed decisions to navigate safely and avoid collisions.
3. Medical Imaging
In the medical field, bounding boxes help delineate areas of interest within imaging data, such as tumors in MRIs or CT scans. This precision aids in diagnosis and treatment planning, showcasing how technology can directly impact healthcare outcomes.
4. Facial Recognition
Bounding boxes are integral to facial recognition algorithms, isolating faces from the background. This isolation is crucial for tasks such as security verification and personalized user experiences.
Bounding Boxes in the Data Annotation Process
The process of data annotation is pivotal for training machine learning models. Bounding boxes play a crucial role here by ensuring that the training data is both accurate and relevant.
Key Steps in the Data Annotation Process Using Bounding Boxes
- Image Selection: Choose images that represent the diverse scenarios that the model will encounter.
- Annotation Tools Utilization: Use advanced software tools like those offered by Keylabs.ai to create bounding boxes around relevant objects.
- Quality Assurance: Implement a reviewing process to ensure precision in bounding box placement, minimizing annotation errors.
- Dataset Compilation: Compile the annotated dataset, which can now correctly inform the machine learning model during the training phase.
The Role of Keylabs.ai in Data Annotation
Keylabs.ai offers an advanced data annotation platform that leverages the power of bounding boxes to improve the efficiency and accuracy of data collection. Their tools empower users to annotate data rapidly with high precision, a necessity in today’s fast-paced business environment.
Consider the following benefits of using Keylabs.ai:
- User-Friendly Interface: Simplifies the annotation process, making it accessible even for users without technical expertise.
- Customizable Annotation Tools: Adapt to various project requirements, whether 2D, 3D, or specialized annotations.
- Scalability: Efficiently handle large datasets to meet the growing demands of machine learning projects.
- Collaboration Features: Support teamwork through cloud-based solutions, enabling multiple users to work simultaneously on the same projects asynchronously.
Challenges in Using Bounding Boxes
While bounding boxes are invaluable, their implementation comes with certain challenges:
1. Accuracy and Precision
Inaccurate bounding boxes can lead to reduced model performance. It is essential that each box tightly encapsulates the target object to avoid misclassifications.
2. Data Complexity
In images with complex scenes or overlapping objects, accurately annotating bounding boxes can become labor-intensive and complicated.
3. Training Time
The annotation process can be time-consuming, especially when manual intervention is required. Efficient workflows and robust software solutions are necessary to streamline these processes.
Future Trends in Bounding Boxes and Data Annotation
The future of bounding boxes in data annotation looks promising, driven by advances in AI and machine learning:
1. Automation and AI-assisted Annotation
As AI technologies advance, automating the creation of bounding boxes using predictive algorithms will likely become more prevalent. This automation will reduce the time and labor involved in the annotation process.
2. Enhanced 3D Data Annotation
As 3D imaging becomes more common, the techniques surrounding bounding boxes will evolve to enhance the accuracy of volumetric data representation.
3. Improved Collaboration Tools
With remote work becoming the norm, future platforms will likely focus on enhancing collaborative features, making it easier for teams to work together on complex projects regardless of location.
Conclusion
In summary, bounding boxes are integral to the fields of computer vision and machine learning, driving advancements across various sectors. Businesses like Keylabs.ai harness the power of bounding boxes to enhance their data annotation processes, ensuring that machine learning models are trained on the best-quality data. As technology continues to evolve, bounding boxes will remain a fundamental component in shaping the future of AI, enabling machines to see and interpret the world as we do. With the right tools and methodologies, businesses can navigate the complexities of image data annotation and leverage these innovations for a competitive edge in their industry.