Labeling Tools for Machine Learning: Enhancing Your Data Strategy
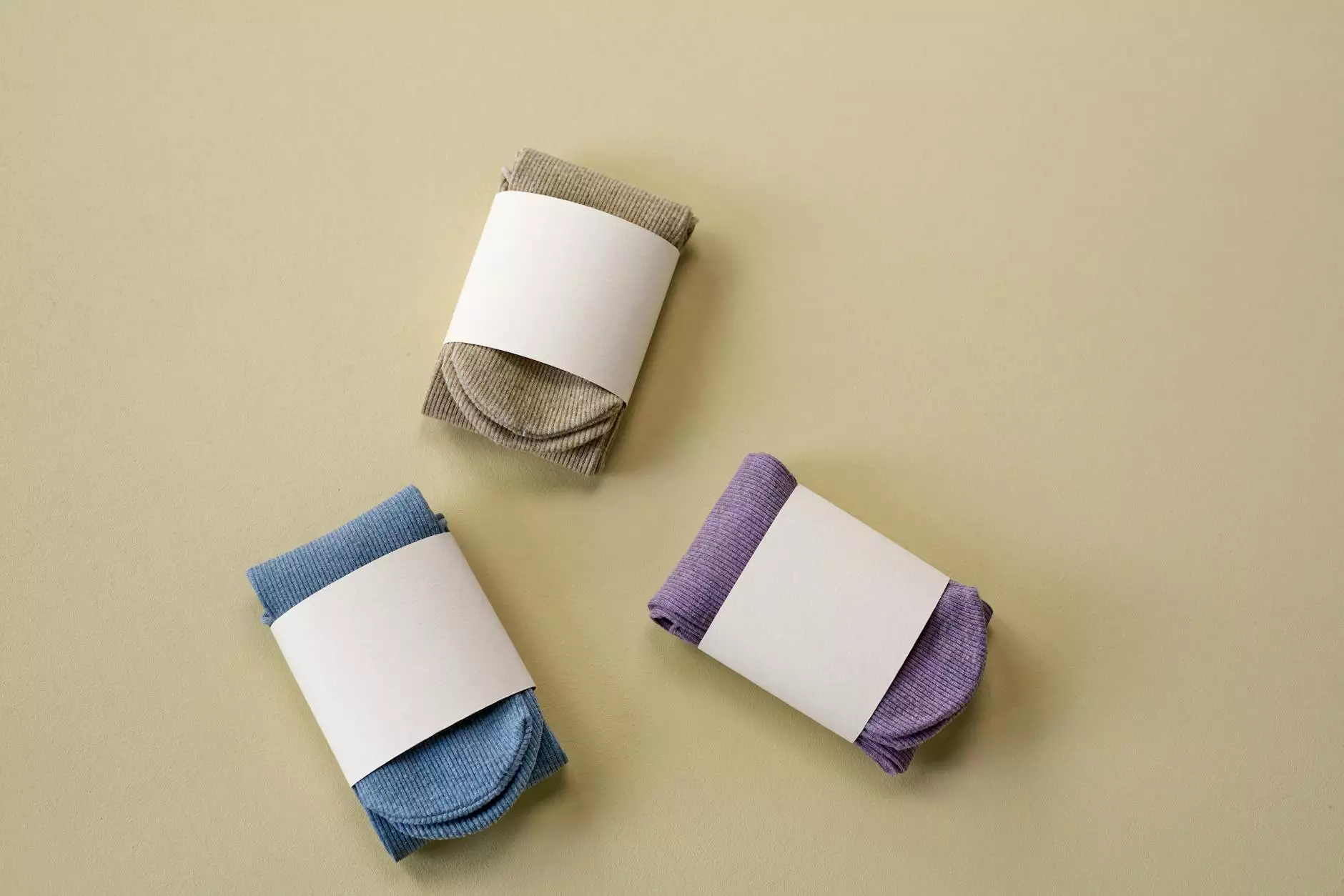
In the realm of artificial intelligence (AI) and machine learning, data is king. However, the quality of your data is contingent upon its labeling. Labeling tools for machine learning play a critical role in defining, organizing, and supplying the necessary data for efficient algorithms. This article delves deep into the significance of effective data labeling and introduces some of the best tools available today. Ultimately, our aim is to demonstrate how businesses can leverage these tools to enhance their data strategies and AI capabilities.
The Importance of Data Labeling in Machine Learning
Data labeling is an essential process in machine learning where data is annotated with informative tags that allow machines to understand and learn from these inputs. Here’s why effective labeling matters:
- Machine Understanding: Without crisp labels, machine learning algorithms struggle to make sense of raw data. Labeled data allows algorithms to generalize and produce accurate predictions.
- Quality and Accuracy: High-quality labeled data leads to better model performance. Insufficient or inaccurate labeling can lead to model errors and poor decision-making.
- Training Efficiency: Proper labels streamline the training process, helping models to learn faster and more effectively.
- Data Diversity: Diverse labeling strategies encourage algorithms to recognize patterns across different contexts, thereby improving robustness.
Types of Data Labeling
Data labeling isn't a one-size-fits-all process; different projects and applications can require specific types of labeling. Understanding these distinctions is crucial for selecting the right labeling tools for machine learning:
1. Image Labeling
This involves assigning labels to images, which is essential in fields such as computer vision. Common annotation types include:
- Bounding boxes: Enclosing objects within a rectangular box.
- Semantic segmentation: Classifying each pixel in the image.
- Keypoint annotation: Tagging specific points of interest on an object.
2. Text Labeling
In natural language processing (NLP), text labeling is essential for sentiment analysis, entity recognition, and more. Techniques include:
- Text classification: Assigning categories to texts.
- Named entity recognition: Identifying proper nouns and their contexts.
3. Audio and Speech Labeling
This includes transcribing audio inputs, identifying speakers, and labeling emotions conveyed through speech.
Top Labeling Tools for Machine Learning
When it comes to choosing the right labeling tool, various factors come into play, including the type of data, project complexity, and team size. Here is a curated list of some of the best labeling tools for machine learning that businesses can take advantage of:
1. Keymakr
Keymakr stands out as a versatile platform serving the specific needs of K-12 organizations, researchers, and enterprises alike. What makes Keymakr particularly effective?
- User-Friendly Interface: Intuitive design that enables quick navigation.
- Scalability: Able to support small projects to large-scale enterprises.
- Collaboration Features: Promotes teamwork with interactive features.
2. Labelbox
Labelbox provides a robust platform specifically designed for image and video annotation. It emphasizes collaboration and integration, facilitating:
- Customizable Workflows: Tailor labeling processes to meet specific project requirements.
- AI-Assisted Labeling: Enhance efficiency with automated labeling suggestions.
3. Amazon SageMaker Ground Truth
Amazon SageMaker Ground Truth offers a feature-rich solution powered by AWS. Its highlights include:
- Integrated Data Management: Effortlessly manage your training datasets on AWS.
- Human Review Capabilities: Combines machine learning with human oversight to improve accuracy.
4. Supervisely
Supervisely excels in computer vision tasks, enabling companies to annotate images and videos effectively. It features:
- 3D Annotation Tools: Useful for more complex object detection tasks.
- Team Collaboration: Multi-user access facilitates simultaneous work on projects.
5. VGG Image Annotator (VIA)
VIA is an open-source project that permits simple image and video annotation tasks without extensive setup. Its advantages include:
- Accessibility: Being open-source makes it accessible to all developers.
- Lightweight: Minimal hardware requirements ensure wider compatibility.
Best Practices for Effective Data Labeling
To maximize the benefits of labeling tools for machine learning, businesses should consider the following best practices:
1. Set Clear Objectives
Defining specific goals for your labeling project is critical. What are you trying to achieve? Having clear objectives helps guide the labeling strategy and ensures everyone on the team is aligned.
2. Choose the Right Tool
Select a labeling tool that fits the scale and complexity of your project. Not all tools are created equal; some are better suited for certain types of data or project requirements.
3. Ensure Quality Assurance
Incorporate quality controls within the labeling process. This includes random sampling of labeled data and having multiple reviewers check important annotations.
4. Promote Collaboration
Encourage teamwork among data scientists, ML engineers, and labelers. Collaborative environments foster open communication and quicker problem resolution.
5. Iterate and Improve
Data labeling should be an evolving process. Collect feedback and utilize it to refine your labeling strategies over time, ensuring improved accuracy and efficiency.
Conclusion
As machine learning continues to evolve and play a pivotal role in business applications, the importance of effective labeling tools for machine learning cannot be overstated. By leveraging tools like Keymakr and others mentioned in this article, organizations can automate, streamline, and enhance their labeling processes, ultimately leading to more accurate and reliable machine learning models.
Investing in the right labeling tools is not just a technical decision but a strategic one that can significantly impact your organization’s ability to harness the power of AI. Undoubtedly, the future of machine learning is bright for those who commit to quality data labeling practices today.
For more information on optimizing your data strategy and exploring the potential of AI, visit Keymakr.com.